Inside Tribe Capital: Building a $1.6B Data-Driven Venture Capital
Zypsy
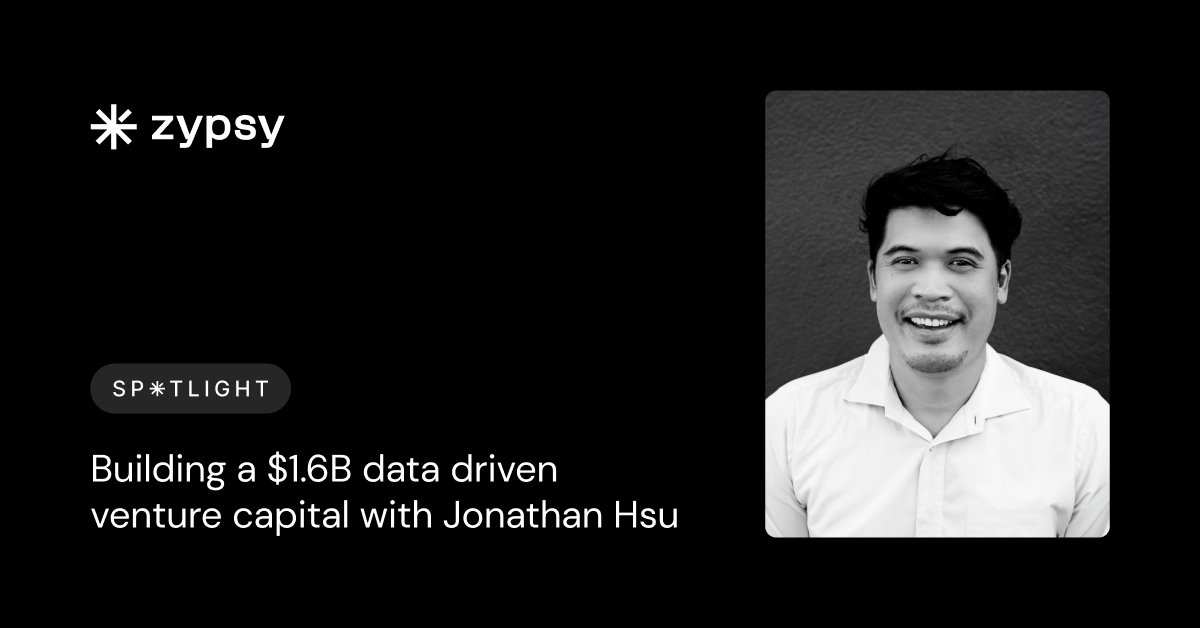
In this episode, we talk to Jonathan Hsu. He is the co-founder of Tribe Capital, a venture capital firm that focuses on using data science to get an edge in venture. Prior to establishing Tribe Capital, Jonathan was the one of the first members of Facebook's data science department.
Jonathan describes his journey from becoming Facebook's first data analyst to co-founding a data-driven venture capital firm, what product market fit accounting looks like, and Tribe Capital's unique approach when founders come to seek investment opportunities.
Subscribe to Zypsy Spotlight wherever you listen to podcasts to learn more useful insights on what the future of venture capital looks like.
00:00 Introduction
01:09 Jonathan’s experience
02:57 New accounting principle for startups
06:02 Quantitative approach to product market fit
08:52 Using the quantitative strategy with Docker
13:02 The history and evolution of accounting
18:16 Future of Tribe Capital
[00:00:00] Jonathan: In venture capital, the most common model is team and market. Almost all VCs say team and market is what matters. And that is a very good model. Don't get me wrong .The point is that you shouldn't have just one model. Things that look like they make no sense in one model, but they make a lot of sense in another model that you wouldn't think to apply. We in particular developed one model and have shared it with the world. And that's where the interesting stuff happens.
[00:00:21] Kaz: Welcome to Zypsy Spotlight. I'm Kaz, co-founder of Zypsy. Design and investment firm that support startup founders with brand building expertise. In this series, we'll be exploring new form of venture capital interviewing from four leading founders and capital allocators in the venture ecosystem. Sharing their insights on what the future of venture capital look like. And today we're thrilled to have Jonathan cofounder of Tribe capital. A VC firm with over $1.6 billion asset under management. And unlike traditional venture capital firm. There are known for developing data driven approach to inform their investment decisions.
And the reason why I'm excited today is not only their unique approach. But also their vision to establish the new accounting standard to measure product market fit for the entire startup ecosystem.
Let's deep dive in. We're cohosts. I'm Kaz.
[00:01:07] Kevin: I'm Kevin.
[00:01:08] Kevin: To kick things off. Jonathan starts by outlining his career path from data scientist to founder and now investor.
[00:01:15] Jonathan: I grew up in Canada and in California and Texas and ended up studying physics at UC Berkeley as an undergraduate, and then did my PhD at Stanford. In the early two thousands, my PhD was in theoretical physics.
Towards the end of my PhD, I didn't wanna be an academic. So transitioned in technology. I spent a little bit of time at Microsoft and then ended up co-founding a small social gaming application that we ended up selling to another company run by Max Levchin, founder of Affirm and PayPal. In between those two companies, he ran a company called Slide.
And that company acquired us and I went to run data for him for a couple of years. After doing that for a little bit, I ended up joining Facebook from 2009 to 2014 and really helped to form and lead that whole organization.
By the time I left in 2014, there are a couple hundred people doing data science at Facebook. About half of it reported to me at the time. And so did that, for about five years. And, by the end of that time, was interested in investing in startups and finance, and had some friends at this firm called Social Capital. A venture firm in Palo Alto, founded by another ex Facebook executive named Chamath. Went there for about four years as a partner, really exploring all the different ways we could use data science in venture. And tried all these different versions, ended up finding a couple of core strategies that worked out pretty well.
And then in 2018 we decided to spin out and form our own firm, so formed Tribe Capital in 2018. And five years in now at this point, we had these early ideas back then, but build 'em to their full fruition here at Tribe, where an entire firm centered around the idea of being able to be experts, growth and product market fit and, and implement that expertise through the data science that we do.
And use that as a tool to invest in early stage companies.
[00:02:56] Kevin: Next up let's hear Jonathan talk about his new accounting principle for startups but first we asked him where he got the idea from?
[00:03:03] Jonathan: The idea there really comes from this broader history of the relationship between analytical thinking and business building. If you look at sort of the long history here, I really think of the first person to use data as an investor is really being Benjamin Graham. About a hundred years ago, when Benjamin Graham was investing, his insight was, prior to him investing was all kind of like rumors and what we would call FOMO now. I believe there's a different way of thinking about value in these companies, and I think I can recognize it using this analytical technique. Those bean counters, you, they call it accounting. And so he was like the first guy to do it. Oh, obviously everybody would be doing that.
But in indeed, everybody was not doing that. And he was rather unique in this case. And so he was the first person to really bring, accounting systematically into investing. To equities. And, and so he did that in about a hundred years ago and that sort of birthed this whole approach of fundamentals investing, which has really been the dominant paradigm for the last hundred years.
And then, fast forward to sort of the mid two thousands. Around the mid two thousands was when we sort of had this big explosion of big data. Prior to the mid two thousands, it was really hard to store and compute on very large amounts of data. It was just, Like logistically difficult.
It was expensive. The technology wasn't there and then all of a sudden in the mid two thousands it was there. It was natural for companies to start using this to both understand their own companies as operational folks, but also people started really using it on the investing side.
Now to be clear, people had already been doing some form of this on this, or quant hedge fund side in the nineties. But, looking at it from the point of view of Silicon Valley, it really didn't hit its full stride until about the mid two thousands. And so, we developed all these analytical approaches.
At places like Facebook and a lot of other companies, and there was a common thread amongst it. A common set of frameworks. And it looks kind of like accounting in some ways, but it's not, it's not the same as accounting, but it looks kind of like accounting. It's different data.
It's not just dollars, accounting is usually like on financial data, but of course, a part of the data that was being analyzed across all these companies back then was really engagement data. web traffic, this type of stuff. And so the question then becomes, is there a standard way to sort of analyze this?
It's kind of like Gap. Gap is sort of a standard way to do it. Now, Gap is a little different in the sense that gap has an external body, that that sort of enforces or creates standards. And in the case of analytics, there is no external body. It's sort of just the ecosystem kind of does it on its own.
And so, what we proposed was sort of a way to, to analyze this stuff in a standard way. Both, that's useful both for operators and, and for investors. I think that's really the key bit. I think it's an important thing to recognize about accounting, for a vast history of accounting the purpose of accounting was more from the operating side, not the investing side.
People used it as operators. Only about a hundred years ago, people used it for investing. And the same way, all this data science, analytics, the primary use case is internal, like product development, business strategy, operations. And it's only recently become useful on the investing side.
And, it's not that it's not useful before, but it's more just that there's appetite from investors who use it.
[00:06:00] Kevin: The next topic will go over jonathan's quantitative approach to product market fit. We dive into this further.
[00:06:06] Jonathan: One of the things that technology has given us, is that it enables you to build a business with a whole bunch of. Engagement or something, we call it product market fit now, but you can think of it as something that comes before revenue.
When you think about the income statement, you usually think like revenue, cost of goods sold, which leaves a gross profit and then a bunch of operating expense. But before there is revenue, there is something else.
And back in the day, 200 years ago, a hundred years ago, you made widgets.
There was nothing before revenue. It's like you make a widget, you sell it. But now, in the age of the social web, you could build software and the software could create engagement even though there's no revenue. And in some sense there's that something, some form of product market fit that comes before revenue.
And so the question is how do you analyze that, that thing that is before the revenue and that was, that's kind of how we think about it. It's important that it comes up to technology because without the modern internet, it doesn't really make sense to talk about something before the revenue in some sense. It's fundamental basis. You can think of it like if you go back to accounting, in some sense, what accounting is the underlying data set is a ledger. A ledger is like, on this day, the business had a cash inflow or outflow to this customer. And then this is a dollar amount, sort of a, either a debit or a credit to the ledger. Something like that. That's what a ledger looks like. And that's the fundamental data set.
And then accounting is sort of like turn that into a standard form. The standard form is an income standard. So turn that into an income statement. The new fundamental data set as of 15, 20 years ago is the, engagement log. It's loosely like an impression log or a click log or something like that.
There's no dollars. It's a customer ID. It's a time or a date stamp. And then there's maybe this is what they did. They clicked this button. They sent a message. That's the fundamental data set. The fundamental data set's very big. Now the question becomes, what's the standard way to analyze that?
And so basically there's like loosely some way of aggregating it along the users loosely. The user ID is loosely some way of aggregating it along the first time that you saw the customers, this is what we think of as cohort analysis. There's something about the concentration of the behavior by customers.
So there's some way of analyzing the concentration. There's this idea of, oh, is the overall thing growing? And if the overall thing is growing, is it, is it growing? There's different patterns of growth. You could just add volume or you could churn a bunch and add a bunch. How do you have a consistent definition of churn, expansion, contraction, and how do you consistently define all these concepts so that you can use them on this generic dataset?
That's kind of the idea, is to have systematic definitions. Just like in accounting, you have a systematic definition of cogs or a systematic direct definition of gross margin or a systematic definition of contribution margin. There's systematic definitions that sort of work. In almost all use cases in the same way.
The goal is to have a systematic definition of these other concepts that we colloquially use all the time.
[00:08:50] Kevin: Coming right up Jonathan sheds light on using this quantified strategy with this specific example in one of his portfolio companies.
[00:08:58] Jonathan: To give you an example, we're large investors in Docker. Docker's a great company. It's been around forever. I think that, depending on your audience people might think of it as the provider of the open source software.
And that's totally a thing. There's this other lens of it, which is as a company that investors invest in. The history of Docker is that the company is very old. It's 13 years old or something like that. At this point, it's first nine years of life, it consumed something like $300 million of venture capital.
This is, you can just see this on Crunchbase consumed an enormous amount of venture capital and they built all this stuff, but they didn't really get to the point where the sort of the underlying business, the revenue base. Sort of the cash dynamics of the business was making sense. And so the company ended up actually getting recapped back in 2019.
And that recap was led by benchmark. They basically did a recap series A and after the recap, they were in this, the company's in this position well, Okay. They've recapped it. It all makes sense now. But, there are all the other investors in the Silicon Valley, everybody's lost money on Docker.
Because they had so many big name investors in it. And so there's this question who would step up to do this this round? Like, who would appreciate what's going on? And, and so from our point of view, we knew all that history. We knew that they had lost all this capital.
They spent all this capital in the past. But using the data, it was able to help us understand sort of that underlying developer engagement in a systematic way. Understand and be able to analyze, okay, they've made changes the way they do the go-to market, they've made changes in the product. Can we actually analyze the efficacy of those products?
See what's really working and basically build, you can think of it as building a narrative that's different from the overall narrative of, oh, they burned a lot of venture capital. And you need to build a separate narrative. And then the question is, once you build those two narratives together, And look at them relative to each other.
Do you arrive at sort of a different conclusion? And we arrived at a conclusion we should, that we would wanna lead that round. So we let the series, the recap, series B of Docker. This would've been was it early 2021. And that company's gone to do super well. They've raised a bunch of extra rounds.
They've grown, phenomenally. But it's one of those things where I think about what was the purpose of the data? All this analysis, the purpose of it was to just control bias. If you go back to when we saw it, when everybody else was looking at it right after the recap there's a bias. The bias is, this company's consumed all this money and didn't get, didn't get too far.
And now it's not wrong. It's a true fact that they did burn all that capital. But the question is, can you come up with something counter to that to sort of give, counterweight that so that you can, have something pushing back against your bias. And that's the point of the data.
The point of the data is to give you something that's basically completely objective. It's just computing the data. Here's the numbers, here's what it is. And like, in your mind, you will create some form of narrative as to what that means. And then hopefully, in some cases that narrative ends up being completely aligned with your preexisting bias.
In some cases it's actually completely different. And that's, and that's in some sense where the interesting stuff happens. The interesting stuff happens when. You have another lens that gives you another view? I'm actually a big believer, broadly as an investor. I'm a big believer in Charlie Munger, Charlie Munger, the great investor, at Berkshire Hathaway, he writes a lot about having many mental models.
It's important to have a lot of mental models. That's what he writes about a lot. He gives a bunch of examples, like there's an economic model, there's a model of, moats and, returns to scale. There's all these different models he thinks about.
The point is that you shouldn't have just one model. His point is that you should have a lot of models because usually the interesting things are things that look like they make no sense in one model, but, but they make a lot of sense in another model that you wouldn't think to apply. And that's where the interesting stuff happens.
For us this quantitative thing is, it's a model of thinking about the world. But it's not the only model. There are a lot of other models. For us, the interesting stuff happens. When the model is kind of all point in different directions, just to give you a sense of a common model.
In venture capital, the most common model is team and market. That's the most common model. Almost all VCs say team and market is what matters. And that is a very good model. Don't get me wrong, I think that's a really important model for VCs. But we have another model . This like very objective product market fit thing.
And, and we use that to counterbalance the team and market stuff because you, we wanna have a lot of models at the table. We in particular developed one model basically from scratch and have shared it with the world. But that's kind of how it fits into investing in some sense.
[00:13:00] Kevin: Hear how Kaz and Jonathan discuss how evolution of technology changed the way we measure startups through accounting.
[00:13:07] Kaz: What I found interesting in your article is that the concept of MAU monthly active user what's popularized by the growth of Facebook. And similarly MRR monthly recurring revenue. Became famous by day immersion of software as a service such as Salesforce. Which eventually made MRR a standard way of discussing subscription revenue. The question is what would the future of growth accounting looks like.
[00:13:34] Jonathan: If you go back to the two, you mentioned, monthly active use and MRR. These are great examples of what tech, this fundamentally technology. Before technology, could get to the point where you could count monthly active users.
It wasn't a number. In the nineties, nobody counted monthly active users. It wasn't, 'cause people didn't think of it, it's because it was computationally, technologically infeasible and impractical to compute it all the time. So people instead looked at page views because page views you could count.
Whereas monthly active users was difficult. The reason why it was difficult, just to be clear, people, your audience may not know this because they might be too young, but like in the early two thousands, if you had like a massive file, like tens of gigabytes and you wanted to count the number of unique ideas in that file, it was really hard.
This sounds crazy now, but back then it was hard because like, it just, the machine couldn't do it. It couldn't, it didn't have enough memory, to like hold all the unique IDs at once, and so it was impractical and so because it was impractical, people used page views. Then it suddenly became practical to do it.
And so you did monthly active users, and that was sort of a, it became a thing. In the same way when you look at MRR. MRR is something you need technology for. The idea of being able to efficiently collect a monthly payment from thousands of customers at a time, you need technology.
If you don't have technology, it would be very impractical. To collect all of that revenue. So you need technology to exist. To give another example. If you look at sort of the sharing economy, it made like GTV a thing, gross transaction volume.
If you think about the business model of Uber or Airbnb, they have this number that's kind of like the gross transaction volume, and then their net revenue from all of these transactions. It's important that their technology is what enables them to even see all those transactions. They have this huge technological base.
At some level, it exists so that they can like pull down all this information on the transaction. If I think about sort of this last phase, maybe the last few years in the forward years, let's look at another thing. One interesting model is crypto. Crypto created this weird form where you could all of a sudden see every transaction of everybody.
There's sort of this mechanic where the protocol will create its own, create new tokens and sort of incentivize folks and you can all of a sudden see all this stuff, and it creates this sort of token economics language. And there are a lot of different ways you can measure token economics into a bunch of standardized metrics.
People have done this right here are some standardized metrics to measure protocol. Interest in this obviously has gone down a bit over the last year or so, but there's a bunch of candidate ideas there and it'll be interesting to see how they play out.
When you look at something like AI.
One thing people talk about is sort of like the value of the training data. Maybe there's some standardized way. To measure both the scale and quality of the underlying training data in the, in the models. And maybe that's a way to understand the value of different companies or different products that are building on top of AI.
Then you run into this question of, okay, is there a standardized way to measure. The quality and scale of the data, there, you could come up with some ways, but it does, it's not so clear to me right now that there is sort of a generally accepted method.
But I think that there's, there's, there's ideas here. In some sense that's the question. The question is what are the ways of measuring it? But it's not just coming up with an approach to measure it. To be clear. Coming up with a way to approach to measure stuff is not hard. There's innumerable PhDs and people with math degree, people like me who have all these crazy quantitative degrees who could come up with ideas in terms of how to measure things.
That's not hard. The difficulty is finding a way to measure things that a lot of people agree on. It's more the social engineering problem of, okay, how do we get people to agree. That's actually the far more difficult thing than coming up with an idea. When I look at sort of the social web quantities, the stuff that's come out of the social web, these concepts are MRR.
In some sense, the only way to get people to agree is to have some sort of financial incentive. In the case of Gap, in the case of the history of Gap, the financial incentive actually comes at some level from the SEC. It comes from like regulatory bodies that exist and say, you have to define it this way.
And so there's a threat of fines. There's a regulatory threat, and there has to be something pushing. In the case of like MRR, the definitions of MRR, all these things, the push comes fundamentally from investors because there's a capital market that wishes to buy the equity.
The capital market says, arrange yourself this way. SaaS companies and SaaS companies arrange themselves this way so that SaaS investors, the incentive is that you'll be able to sell your equity to these investors. And so in the same way, when I think about this, the future of, sort of how we think about AI, how we think about crypto, these ideas at some level, It's a question of how should those ecosystems arrange themselves to make themselves interesting to potential investors to the capital markets?
And the capital markets. There's sort of this back and forth between the capital markets and the, and the founders and the innovators in terms of what's the language we're gonna use that both sides feel comfortable with.
[00:18:14] Kevin: The last question we asked Jonathan was what does the future of Tribe Capital look like?
[00:18:19] Jonathan: In our model, the important thing isn't just that we do the data, it's that we give away the analysis to founders.
When founders come to, to seek investment with us or to work with us. The whole point is, hey, we will analyze your stuff for you and we will give you back the benchmarks all for free. The point being that even if we don't invest in you, hopefully we're giving you something valuable. We're, we're giving you some very detailed be benchmarks on some very precisely defined and measured quantities to give you some of that feedback so that you can use some of that to help you run your own business, so the whole point is for us to give that value back into the ecosystem. There's a component of it that helps us, that means we see more companies. If companies know that there's like something, they get out of it, they have an incentive to come talk to us, even if, even if we may not invest.
And so that's an important aspect of it, is that. What we do differently is that we do this data work, we interact with the ecosystem to help them as much as possible, and then obviously it helps us. It's pretty clear how it helps us. We're not being secretive about it. It's different from like how historical, like quantitative hedge funds do things.
Like historically in quant hedge funds, they have like a data black box, and it's totally secret. They don't share it with anybody. That's not what we do. We give it all give give away. You can go on our website, you can read about how we do it. We literally tell the world this is how we do the computation.
The thing that's special obviously is that we are gonna do it for you for free. From the founder's point of view, that's the special bit. So we have a little model, in which we use the data to interact regularly with the ecosystem to sort of be able to help as many founders as possible. That's our model. When I think about sort of this broader question of like all the forms of venture capital that exist and all the different things that people are doing, the broader phenomena of course is just that there's like more interest in venture capital now than there was 15, 20 years ago.
There's more LPs, more capital that's coming into the market, even though we're in a bit of a draw down right now. For sure. Macro, we're in a draw down. Like the, the overall trajectory is pretty clear. In four or five years we'll be, sort of, we'll be back, at least we believe we'll be sort of back into a similar spot, maybe not as 2022, but certainly back in 2018, 2019 era.
What happens is when you have all of this capital in, there's two effects. On the one hand, you'll get these big mega funds, which we know about. And they dominate the news headlines. But there's this other side of it, which is that there's a whole bunch of different investors.
Because what happens is when all the capital comes in, different capital wants to do different things, different cap. This LP might think, oh, I think the way to invest is like this. I wanna find a venture investor who does X, Y, Z for me. But then there'll be some other LP who's like, whoa, wait, I, I think it should be something else.
I think what matters is A, B, C, and then there'll be a manager. To give them that. So I think of this like massive flowering of investment managers and investment strategies. As being sort of a necessary side effect of all of this, this capital coming in. And fundamentally for founders.
That's good. Because if you have all these managers, if you're a founder, that means you have like many more investors you could talk to. And a better odds of being able to find a couple that really align with what you're doing and who could be good partners for you as a founder. So I think that's sort of the macro thing that's going on.
Will there be more data in venture? Well, yes, there'll be more managers that use data like us. But will there also be more managers who don't use data? Yes, there will be both. Everything will happen. Because that's sort of the nature of this stuff. There will just be more variants because there are more things that capital wants to do.
This system doesn't really consolidate the same way that like fixed income, ETFs consolidate even that consolidate. But this doesn't have a tendency towards consolidating because there's this sort of level of engagement with the underlying assets.
There's this level of, What is it that people wanna get out of these investments? Part of it is certainly financial returns, but to be clear, for a lot of LPs, it's some, oftentimes something else, they wanna engage with the ecosystem. They wanna be a part of a story, and if the LP wants that, they can find a manager who will implement that for them, and that will help those founders come into existence.
[00:22:02] Kevin: If you liked this Spotlight episode, please leave us a review. We're just starting out, so every review really helps. Follow us on Twitter at zypsycom if you don't want to miss an episode. That way, you'll be able to see every time a new show goes live. That's all from us today. Thank you for listening to this episode of Zypsy Spotlight.