Inside Zetta: The rise of AI-driven bio companies
Zypsy
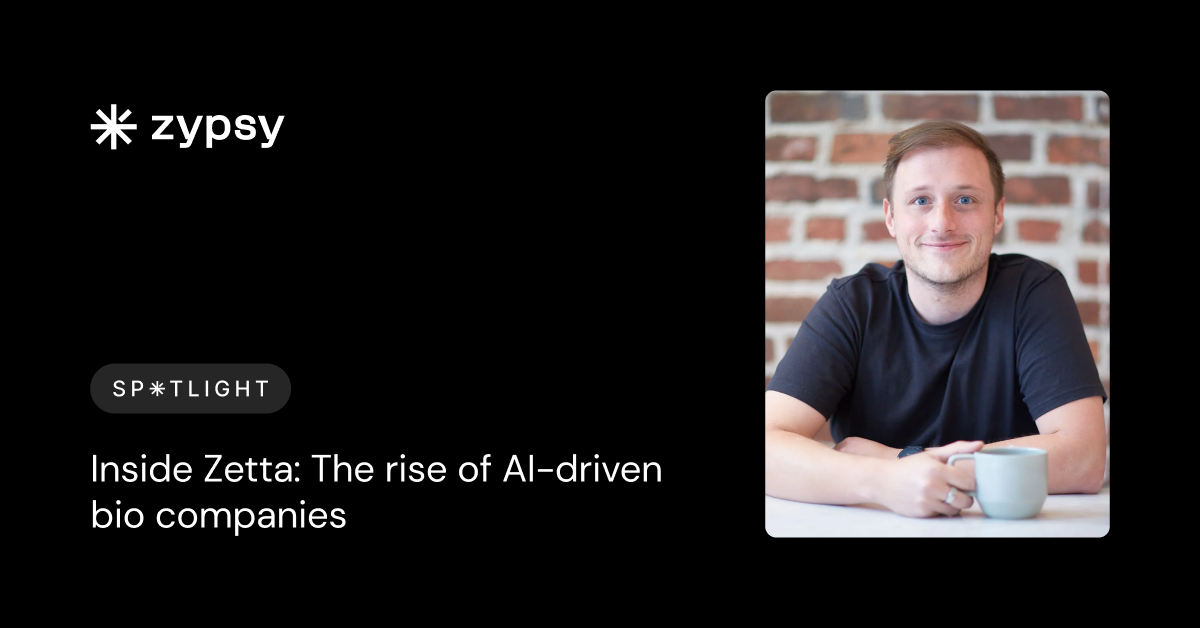
In this episode, we talk to Dylan Reid. He is a partner at Zetta Venture Partners, the first VC firm exclusively focused on identifying and supporting AI-driven, B2B businesses in critical domains like biotechnology, climate technology, DevOps and cloud infrastructure, security, and more.
Dive into Dylan’s thoughts on the convergence of AI, biology, and business, understanding the evolution and future prospects of AI startups. He reflects on Zetta's dedication to fostering AI solutions, emphasizing the importance of practical AI applications in advancing biology and healthcare.
Subscribe to Zypsy Spotlight wherever you listen to podcasts to learn more useful insights on what the future of venture capital looks like.
00:00 Introduction
01:00 Dylan’s journey
03:27 LLMs and biological data
05:54 Advancement of technology
09:55 Finding and interacting with biotech founders
13:47 The power and promise of data in drug discovery
20:24 The world of research investments and potential breakthroughs
[00:00:00] Dylan: Just starting to happen now, and I'm really excited about is the diaspora of people from the first generation of AI driven bio companies that have gotten to scale.
Those companies took in people from biology, CS, labs, and built really the first industrial scale data generation platforms for machine learning.
[00:00:22] Kaz: Welcome to Zypsy Spotlight. I'm Kaz, co founder of Zypsy, a design and investment firm that supports startup founders with brand building expertise. In this series, we'll be exploring intersection of AI and biology. ChatGPT learns to talk by reading lots of internet texts. Midjourney learns to create unique images by analyzing billions of them.
So if the large language model studied biological data, what opportunities do they unlock for the future? Today, we're excited to have Dylan, a partner at Zetta Ventures, investing in a number of digital biology companies that have gotten to scale. Let's deep dive in. We're your co hosts, I'm Kaz.
[00:01:00] Kevin: I'm Kevin.
Through the lens of his early days at MIT to his role at Zetta Venture Partners, Dylan sheds light on the growing importance of data and machine learning.
[00:01:10] Dylan: I'm one of five partners at Zetta Venture Partners. We're an early stage funds for technical founders for building products around data and machine learning. My partner, Mark started the fund 10 years ago at the very beginning of what we can think of as the modern era of deep learning, this is just as ImageNet had been won by the team at the university of Toronto, and it was clear that, software was getting easier to build and the real power from technology was going to come from data and the kinds of algorithms that we could build around that.
We're typically the first institutional investors to back founders often at inception stage. We're usually leading or co leading their first round. And we really try and be active partners to our investors. We make many fewer investments than most funds of our size, somewhere between 20 and 25 per fund and instead of 180 million fund, we reserve a lot more capital for follow ons. And, we're making a commitment to founders, usually when they're just at the idea stage with the deck and and some ideas that we're going to help them build a company and get from zero to one.
And we take a really boutique approach. Every company is different. And so we don't have a platform team. We don't have a big investment team. It's really the five of us working closely with each of the companies that we support and the companies that we support across the portfolio. My background is in ML as well.
I was lucky enough to see the very early innings of deep learning working computer vision at around the same time that Mark was starting the fund. But I was over at the media lab at MIT. Spun a company out of there and manufacturing space, which was really one of the first applications of machine learning for enterprise. Thinking about how to make manufacturing more efficient. And that was about 10 years ago and have really not looked away since. So spent the rest of my career truncated by building a company and then joining Zetta to invest in companies all around how you leverage machine learning to build things that are really new and interesting and important. For the last couple years I've been really transfixed by applications in biology and health both because I think they're really important and meaningful.
But also I think it's where machine learning is gonna have the biggest and most nonlinear impacts where things I think in the future will look very different as a result of these technologies than they do today.
[00:03:27] Kevin: Up next, Dylan gives a clear picture of the huge possibilities that lie ahead as he explores the link between LLMs and biological data.
[00:03:34] Dylan: Today we've got large language models and large self supervised pre trained models that are learning, directly from biological data from protein sequences and DNA and RNA sequences all the way up to human tissue.
The same way that these large language models like ChatGPT, read all of the sort of comment sections and every wikipedia article all the sort of textual data on the internet. These models are able to learn from these big databases and experimentally derived libraries of biological sequences and rather than learning, you know the context of how language works meaning semantics all the things that go into really understanding language and having cultural fluency or conversational fluency these models are learning a lot of the underlying, pinnings of how, structure and function emerge from biological data like sequence data for proteins.
So using that basically internal representation of what they've learned which I think is important to say, we don't fully understand, the way that we understand human language. We do not have the same grasp of biological language, but these models are building really rich internal representations of that and can use that basically for a whole bunch of downstream tasks, some of which are generative.
Using the ChatGPT analogy, instead of generating a poem or a response to a question as a prompt in natural language, they can generate functional proteins. Sequences that can be synthesized, that can be used as drugs, as new materials, as biosensors for diagnostics, using that sort of same analog.
Amazingly, this this seems to be working working really well in the protein space. We have a company there and there's quite a few others that are, building these sort of ChatGPT like products for various proteins and then, using the output of those to develop drugs, to develop materials.
And we're starting to see it scale to other modalities as well. There's protein language models, there's DNA language models, there's now people working on mRNA and RNA language models, and also, push the boundaries on what's historically been possible in these fields, given how little we understand the sort of functional relationships of all these really complex molecules that dictate life.
[00:05:54] Kevin: Drawing from his extensive experience and current projects. Dylan provides a clear snapshot of where technology stands now and his trajectory for the future.
[00:06:03] Dylan: It's the collision of two forces, which is the data generation and the computational technologies are moving extremely fast. And drug development is extremely slow for good reasons, right? If you're going to put something in, thousands, millions, even billions of people, if you're going to permanently change their biology in some meaningful way, you really want to make sure it's safe.
And given how little we know about human biology, the only way to do that is by testing it exhaustively, in people in a very controlled environment, so clinical trials. The big bottleneck, I think, has, and will probably always be, clinical trials. But in terms of what we're able to do and show in, petri dishes in the lab and increasingly in mice and larger animal models is really profound and really interesting.
There's a couple of big themes in our portfolio at this intersection. One is in line with the shared with the next protein company. I talked about sort of rational molecule design. Can you take a lot of traditional discovery processes where you used to, mine through large libraries and what chemicals and test them to see if you can find something to starting with what you want, whether it's a particular target or a particular set of characteristics and design that molecule from scratch. I think what we're seeing there, especially in the biologic space, that's, protein based drugs like antibodies, it's gene editors, it's transient editors like mRNA. I think what we're seeing there is one, the ability to design these molecules in a really precise way , but rational design paradigm is letting us do things that would have just been impossible or would have been very difficult in the rational or in the discovery world.
A good example of that is we have a company Nabla, which is taking sort of known antibodies that, are able to hit certain targets, but are not developable or not manufacturable, or basically not, they work in a lab, but they're not viable in production. And they're able to really dramatically change the properties of those so that they're, highly stable, so that they're less immunogenic, so that they have these properties that make them viable and easy to administer.
I think we're already starting to see the next generation, which is not only, how can we make existing drugs much better, but how can we use these biological substrates? Antibodies, non antibody, scaffolds, mRNA, to do new things. Whether to hit new targets that were previously thought to be undruggable whether it's, affecting multiple targets at once that are bispecific or polyspecific.
That have changing characteristics once they get into the cell or once they're in the presence of a certain kind of protein, change the way that they interact. These are things that are totally new and totally weird and I think have the potential. This stuff takes a while and there's still so much we don't know about biology, but they have the potential to really change medicine. To address, new intractable diseases,to dramatically change response rates. We have a lot of drugs like in immunotherapies that work extremely well on a very small percentage of patients that they're given to. And can we build those out for every new subtype and all kinds of new things. That's an human health and disease. There's obviously a lot of people interested in, tackling longevity and aging, which is the inverse of the disease paradigm.
And there's a lot of applications outside of human health as well. We're seeing some really interesting things happen and applications for alternative energy sources, inputs to, biofuels and things like that. We're seeing industrial consumer products, things like alternative proteins and meats and all kinds of things, building materials.
I think our work has been much more focused in on human health, but it's clear if you can rationally design biological building blocks of the world, they've got applications that are much broader.
[00:09:55] Kevin: Building on his insights from Zetta Venture Partners, Dylan goes into more detail about how to find and interact with founders in the field of biology.
[00:10:03] Dylan: The number of people that can start a software company and get it to scale, it's just, orders of magnitude bigger than the kinds of people that are going to be successful building these companies. Given that there's actually a very small world of potential founders, we take a kind of inverse approach, which is looking for the pockets of people that are most likely to have the unique insights and the tribal knowledge to build these companies and then figuring out what they're excited about, what they're working on. And a couple of high level things we're really looking for these like deeply intersectional teams.
We call them bilingual or multilingual teams. Not biology teams, not computational teams and not teams that have one computational founder and one biological founded that exist in silos. The thing that we've seen really work in this space is the deep intersection. These are computational people that really understand.
The underlying biology understand the experimental methods, and these air experimental biologists that really understand data and how machine learning and the reinforcement loops work. It's only in that intersection of people talking to one another. Those two pieces are really intractable from one another.
The world of people that are truly bilingual in this space, again, is really small. And it tends to be, I would say, two big buckets. The first is, you mentioned labs, there's, I would say, still a pretty small number of academic labs that are really at this intersection. Now, these are places like The Baker Lab at University of Washington, George Church's Lab at Harvard, and the Brangham's Lab at the Broad Institute.
I would say maybe two dozen more. And these are places that are not bio labs. They're not CS labs. They really are looking at that intersection, developing new experimental techniques for machine scale learning. And vice versa. We're just starting to see a new generation of these sort of bilingual researchers, who either learned biology, like through the lens of machine learning or through machine learning with, biological use cases and applications. So that's, very new and a small enough world of those people that we can spend real time with them. We follow research really closely coming out of those labs were there and long before there's a company often were or, building that relationship with them and we're, really aware of kind of the work that they're doing.
What's nice about academia is it happens out in the open, with pre prints. Now it's happening much faster. So a lot of what we do is I would say talking to non companies, talking to interesting scientists that are doing cool stuff. And then the second bucket, which is just starting to happen now, and I'm really excited about is the diaspora of people from the first generation AI driven bio companies that have gotten to scale.
This is, folks from Recursion, Exscientia, Insitro, Immune AI. Those companies took in people from biology, from CS, from some of those labs, and built really the first industrial scale data generation. Platforms for machine learning and the people who are coming out of those companies now, I've seen this playbook I think have learned what worked and what didn't work and come to this problem with a ton of tribal knowledge Which is actually hard to get I would say even in academia just because the scale is so much smaller the kinds of experiments you could run as a multi billion dollar company versus an academic lab are really different and scales, you know Increasingly important both on the compute and the data side for machine learning.
Those are places where actively getting to know folks and and starting to back companies and the rest comes from there. We're doing our job right. And we're being good partners to our founders. We see more and more new opportunities and interesting research through the portfolio versus on our own.
[00:13:47] Kevin: Tracing back to his interactions with the founders of Noetik, Dillon walks us through the power and promise of data in drug discovery.
[00:13:55] Dylan: Our most recent announced investment, a company called Noetik which is working on next generation of oncology drugs. The founders there, Jake and Ron were at Recursion. Ron was one of the earliest employees. A lot of research was the CSO at one point.
And Jake was a good friend of his from college. They went to Stanford together went on to Genentech and Ron recruited him to Recursion a couple of years then to help build out the oncology team. At the time Recursion was really one of the first AI driven drug discovery companies built, probably even to this day, the biggest large scale data generation platform, looking at cell painting and using some other techniques and it was mostly focused on rare disease in the early days.
So they built out this oncology team. It was pretty small, almost like a little skunkworks and grew to be a really meaningful part of their platform. And today I think it's probably more than 50 percent of their focus actually Recursion, just announced some promising results from a partnership with Genentech out of that team.
And Ron had seen, one of these companies really built from the ground up and had as an early employee, had served every role that there was from, building the platform to business development and partnerships and really gotta see, I think the power of.
Large scale data generation or industrial scale data generation. But also some of the limitations of working with cell line data, which is what they were looking at. Not data from humans, but from cell lines that you then perturb in this way. And I think him and Jake, like so many people in the space have, a lot of personal connections to cancer and a real passion for trying to do cancer drug development better. The two of them left to start Noetik with a lot of the insights, like the functional insights that they had gotten from. Recursion but with a different model and some really different ideas based on having started the company six years later and I would say the two big ones are one starting with human data. So can you learn directly from data samples derived from humans. In their case, looking at human tumor samples, and so not individual cells, but entire slices of tumor to see how cells within the tumor interacting with the immune cells, the immune system, this tumor micro environment, so much more complex biology than what in a single cell.
Then using new experimental techniques in their case, spatial imaging a whole suite of technologies that allow you to see not just what's happening in an individual cell, but that cell in its spatial context. So groups of cells coming together to mount an immune response, say to a tumor in order to shrink a tumor, basically.
The first insight was around, can we build very different kinds of data? Can we learn from, richer, more human representations, taken directly from patients? These are not, models. The second. Which I think gets back to this first question about about LLMs was, could you learn in a totally self supervised way?
Could you train something like a foundation model on this human biology to learn fundamentally new insights about cancer that you could then use to develop about drugs. This is just an evolution of having started a company in 2022. They had this insider, really a hypothesis that if you can generate this large human data set, a really rich one you could use that to feed a model.
Think of it as like a ChatGPT model, but instead of, text or protein sequences, which are sequential, could you feed it really rich multimodal data? So images, transcriptomics, proteomics, multiple modalities of what's going on and train a model to learn this like really rich and meaningful representation of immune response. Basically to predict what tumors, so not necessarily even what patients, what tumors are likely to respond to immunotherapies. If you could do that, could you basically use that to develop, new, much more precise immunotherapies, not those that worked in 20 percent of patients.
They were giving it to about closer to a hundred and lots of those almost treating cancer, like a rare disease. If you could do that in a totally self supervised way, you could learn new cancer biology, you could learn you're not beholden basically to human labels, which is obviously really important when you're trying to figure out something that.
You don't understand like immune response. But yeah, so so got to know them, you know early on I'd spoken to a lot of founders out of recursion and interestingly, they all had a lot of the same Kinds of insights applied to different things. Let's generate different kinds of data. Let's use different learning methods.
Let's try and develop drugs faster. A big learning from the first generation of these companies is they just took a really long time and spent a lot of money on the platform before getting into drug development. Talk to people who are working on the experimental side who are really pushing the boundaries of spatial and a couple others and I was just really enamored with the idea I think at a time where certainly before ChatGPT.
I think the image generation tools the diffusion models were just starting to come out one of the things that really gave me conviction that this was possible was playing with early versions of Midjourney and DALL-E and seeing that these models were learning really complex representations of image space, they were able to create images in the style of Francis Bacon, in the style of Picasso and in doing so we're learning something very deeply essential about what those artists did and what they meant without actually being told any explicit instructions and the idea that could work for medical images to unlock new biology was you know on one hand felt like a long shot, but also thought wow if this was possible, it's worth doing and the team that's going to crack it is the team that can generate the right data to train the model and then knows what to do with that model, how to learn from the internal representations, how to validate them and nobody was trying to do this and of the people who are trying to do it, nobody had the sort of expertise in data generation and the biology and the drug development that this team does . Still early, but they built a really remarkable data set in a really short amount of time and they're training their first models that fingers crossed seem to be learning some novel biology that's really meaningful and potentially drivable, these are the moments you live for as an investor or anybody who works with startups.
[00:20:17] Kevin: In this final segment, let's dive into how Dylan navigates the world of research investments and evaluates potential breakthroughs.
[00:20:24] Dylan: As a general case oftentimes in medicine, the problems are well understood and well articulated, and the business case is clear, and so it becomes almost the inverse of product market fit, which is can you build the product?
So there's, a ton of technical and scientific risk. If you choose the right problem area, and actually I think that's really important so if you start with a problem that you know is valuable to be solved and you can actually do it, then I think everything else is a little bit more straightforward.
If you can design an antibody that does x y and z like often the way these partnerships work is like they're really clear on what this the customer is very clear on what the spec is And they'll tell you that ahead of time. So that's good actually doing that is extremely hard and the only reason that I have the confidence to invest in these things is because it is truly unknowable, a priori, what is going to work.
Very few people have sort of intuition for what It's possible at this sort of new intersection of, large scale data generation of machine learning. And so we're all basically starting from zero. And it's a quest to see who could learn the most and the fastest and most relevant with as few priors as possible, I think has been really helpful.
So it's really about trying to develop, I think, intuition for what might work and the kinds of people that that might be able to pull it off. In the Noetik case had some intuition or hope that these sort of like large scale vision based transformers could learn like really meaningful biology from. Multimodal image data had a lot of conviction that team really understood the nature of the problem in terms of generating data in terms of training the model in terms of making something out of it. And venture is about getting it right, like 10 percent of the time in a really big way. And so for starters, you're wrong all the time, that's, I think helpful. In the case like Nabla, they had published some really interesting academic work that was getting some attention.
There was, a lot more to look at and say, hey, this technology looks really promising. And then the leap is, can it be applied to this particular problem? So everybody solves these problems with toy problems in academia, which is useful for all kinds of reasons.
We've cured cancer in millions and millions of mice, but I have a real problem doing it in people. It's a more translational question. But again that's no different in this domain versus any others is at the early stage, you're making a bet on the founders and you're making a bet on the fact that they're going to be able to unlock something really unique and interesting, through a combination of an insight that they have and determination.
[00:23:04] Kevin: If you liked this Spotlight episode, please leave us a review. We're just starting out, so every review really helps. Follow us on Twitter at zypsycom if you don't want to miss an episode. That way, you'll be able to see every time a new show goes live. That's all from us today. Thank you for listening to this episode of Zypsy Spotlight.